Build Responsible AI with AI Observability
Fiddler is a pioneer in AI Observability (formerly known as Model Performance Management) — the foundation you need to standardize LLM and MLOps practices. AI Observability is reliant not only on metrics, but also on how well issues can be explained when something eventually goes wrong.
Fiddler empowers your Data Science, MLOps, Application Engineering, Risk, Compliance, Analytics, and LOB teams to monitor, explain, analyze, and improve model performance.
- Deliver high performance AI
- Reduce costs and increase ROI
- Be responsible with governance
Build Trust into AI with Fiddler
- Unified environment: Provides a common language, centralized controls, and actionable insights to operationalize ML/AI with trust.
- 360° view into AI behavior: Integrates deep XAI and analytics for a complete understanding of why and how predictions are made.
- Enterprise-grade solution: Enterprise-scale security and support without the hassle of building and maintaining in-house LLM and MLOps monitoring systems.
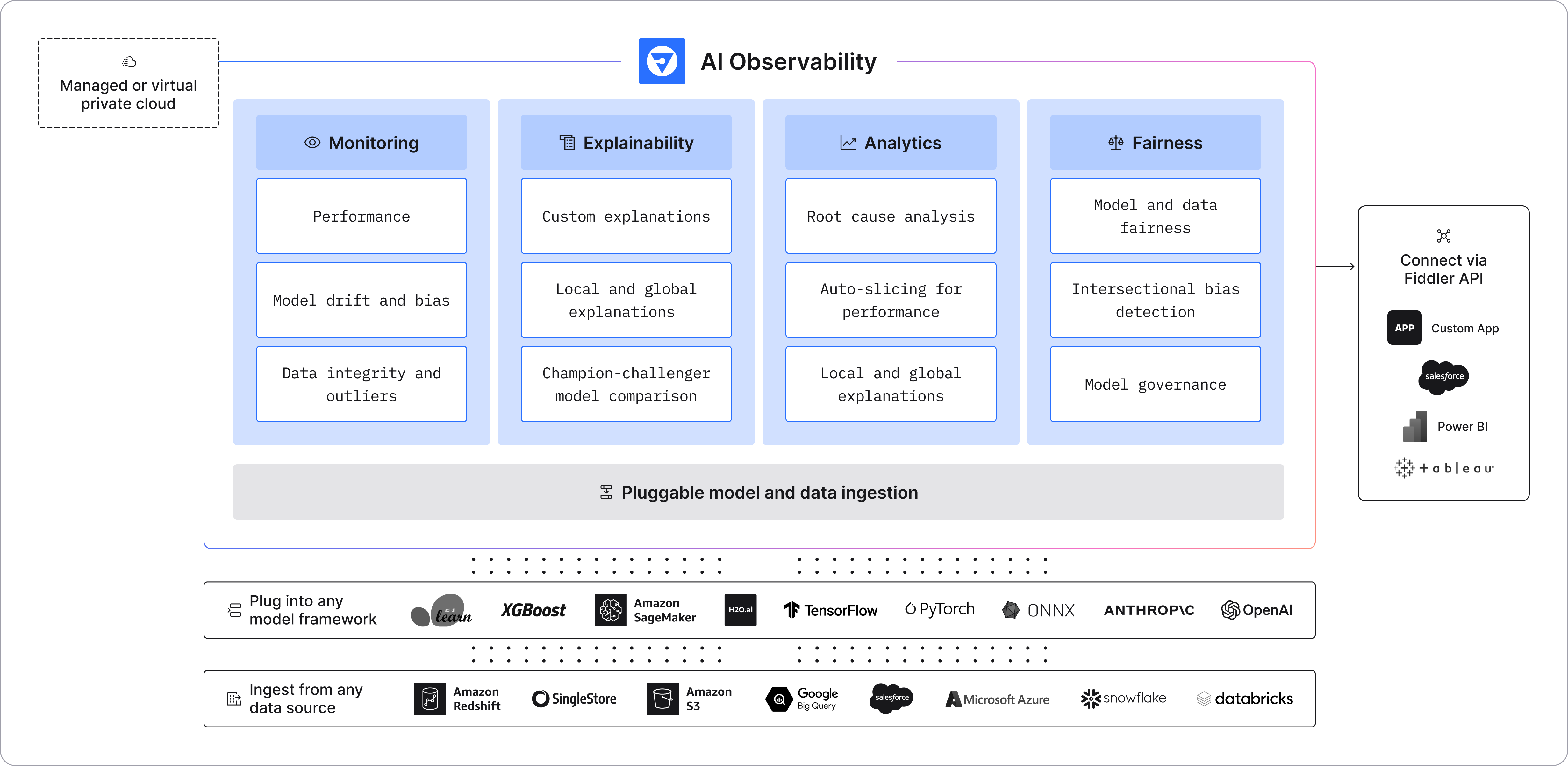
Fiddler understands both the data scientist’s perspective and the manager’s perspective, which is the value we appreciate the most. As a manager, I get the visuals and explainability I need, and data scientists get the model monitoring and other technical stuff they need.
— Amit Attias, CTO and Co-Founder at Bigabid
Key Capabilities
Monitoring
Monitor predictive models and LLMs in pre and post-production and manage all performance metrics at scale in a unified dashboard. From model monitoring alerts to root cause analysis, pinpoint areas of model underperformance and minimize business impact. You can also find quick answers to the root cause and the “why” behind all issues.
Plug Fiddler into your existing ML tech stacks for consolidated model monitoring to:
- Gain efficiencies through faster time-to-market at scale
- Reduce costs by decreasing errors and the time required to resolve issues
- Improve collaboration and team alignment with unified monitoring and silo elimination
Explainable AI (XAI)
Fiddler uses proprietary explainable AI technology to provide complete context and visibility into ML model behaviors and predictions, from training to production. Implement powerful XAI techniques at scale to build trusted AI solutions that help you:
- Maximize confidence through explanations for all model predictions
- Minimize risk by deploying AI governance and model risk management processes
- Increase brand loyalty by delighting customers with responsible AI
Analytics
Analytics must deliver actionable insights that power data-driven decisions. To improve predictions, market context and business alignment must be baked into modeling so results reflect the needs and challenges of your business.
Use descriptive and prescriptive analytics from ML models and LLMs to make decisions so you can:
- Deploy higher ROI models to increase revenue
- Align decisions to stay in lockstep with business needs
- Respond quickly and refine models when market dynamics shift
Fairness
Responsible AI is the practice of building transparent, accountable, ethical, and reliable AI. The first step is detection and mitigation of bias in tabular and unstructured datasets and ML models, but you must also support internal governance processes and reduce risk through human involvement.
Build and deploy responsible AI solutions with bias detection and fairness assessment in order to:
- Reduce risk by instilling trust with continuous AI monitoring and human decision-making with ML
- Provide visibility and governance to internal oversight teams
- Mitigate model bias through the detection, comparison, and measurement of dataset bias
Use cases
- Risk Mitigation
- Anti-money Laundering
- Fraud Detection
- Credit Scoring Investment
- Decision-Making
- Underwriting
- Churn Detection
- AI Governance