Fiddler for Insurance
Insurance leaders are harnessing the power of AI to innovate and grow their business in evolving market dynamics, streamline customer experiences as well as providing protection to customers whenever and wherever they need it — whether it be their health, homes, cars, family, or financial future.

Fiddler is a pioneer in AI Observability and Security — the foundation to ensure the performance, behavior, and safety of predictive models, generative AI applications, and agents. Insurance companies are equipped with a comprehensive AI Observability and Security platform to productionize predictive models, generative AI applications, and agents responsibly and at scale.
The Fiddler AI Observability and Security platform aligns developers, platform engineering, data science, and business teams through the lifecycle to monitor, analyze, and protect models and applications.
Why Insurance Leaders Choose Fiddler
- Unified Environment for GenAI and MLOps: Provides a common language, centralized controls, and actionable insights to operationalize predictive and generative AI with trust.
- Built for the Enterprise: Enterprise-scale security and support without the hassle of building and maintaining in-house LLM and MLOps monitoring systems.
- Expert AI Team for Responsible AI: Fiddler’s expert AI team is dedicated to helping enterprises succeed in their ML and LLM deployments, ensuring the achievement of responsible AI.
Fiddler Supports Advanced and Complex AI Use Cases for Insurance
Generative AI Use Cases
- AI Chatbots: Boost customer satisfaction with accurate and helpful LLM chatbots. Ensure the accuracy, safety, privacy, and correctness of NLP and LLM-based chatbot conversations. Monitor key metrics such as hallucination rates, toxicity levels, and user feedback to maintain highly accurate chatbot interactions.
- Internal Copilot Applications: Enhance employee productivity and boost their confidence in decision-making. Boost your employees’ confidence and trust in information generated by LLM applications for business-critical projects. Fiddler monitors a comprehensive range of LLM metrics (hallucination, safety, PII) to ensure that these applications provide correct and accurate information for financial planning and investment analyses.
- Risk Management: Detect adversarial attacks and data leakage. Detect jailbreaks and prompt injection attacks that risk LLM applications from exposing sensitive enterprise and customer information. Visualize patterns in a 3D UMAP and identify all prompt injection attacks using Fiddler’s Slice and Explain.
- Cost Management: Increase LLM operational efficiency gains. Track the costs of using LLM applications by obtaining a complete view of your LLM costs, latency, and session length. Use Fiddler’s custom metrics to measure efficiency gains, helping streamline processes and enhance productivity.
- Content Summarization: Enhance the accuracy of LLM summarization by monitoring hallucination metrics such as faithfulness, answer/context relevance, coherence, and consistency using Fiddler Trust Models. Quickly detect signs of hallucination, diagnose and address their root causes before inaccurate LLM summarizations can impact the enterprise and its users.
Predictive Use Cases
- Claims Process Automation: Streamline claims process to reduce manual reviews, improve efficiency, and achieve cost savings.Understand how claim models are processing claims, ensuring claims are processed accurately. Discover why certain claims are approved or denied with explanation methods, like Shapley Values and Fiddler SHAP. Drill down on local and global-level explanations to understand how each feature contributed to claim decisions.
- Image Assessment for Claims: Deliver accurate and transparent assessments of claims. Use image-based explainable AI (XAI) to accurately evaluate damages and calculate fair settlements for car, home, and property and casualty claims. Enhance decision-making with insights from model predictions and integrate a human-in-the-loop process to ensure optimal outcomes.
- Fraud Detection: Safeguard the company and customers from fraudulent activities, and reduce the risk of financial loss. Improve the detection of fraudulent claims by closely monitoring subtle shifts in data caused by models processing highly imbalanced data inputs, and get real-time alerts as soon as anomalies come up.
- Pricing Optimization for Premiums and Bundling: Increase the confidence in premiums and bundling of products and services. Accurately assess customer risk to optimize premium pricing and bundle offers. Understand how multi-dimensional variables lead to pricing shifts. Check for model and data fairness to ensure prices are competitive, fair, and transparent.
- Personalized Recommendations: Delight customers with personalized recommendations. Use Fiddler to uncover why segments of customers are offered specific products and services, and which factors contribute to those recommendations. Understand which attributes from segments of customers contribute to product recommendations.
Key Capabilities
Monitoring
Monitor predictive and generative AI applications models across pre-production and post-production. Track ML and LLM metrics at scale in a unified dashboard. Use the Fiddler Trust Service to quickly, safely, and cost-effectively measure LLM metrics with accuracy.
From model monitoring alerts to detailed root cause analysis, quickly identify and address model issues to minimize business impact.
- Metrics Monitoring: Accurately detect changes in model behavior by monitoring ML and LLM metrics
- Drift
- Data quality
- Hallucination
- Toxicity
- Prompt injection attacks
- PII
- Custom
- Segment Monitoring: Monitor segments to drill down on underperforming cohorts
- 3D UMAP Visualizer: Gain contextual insights by identifying patterns and outliers in high dimensional spaces
- Alerts: Configure and receive real-time model monitoring alerts to identify and troubleshoot high-priority issues

Analytics
Gain actionable insights to power data-driven decisions. Analyze slices of data to surface the root cause of issues. Understand the 'why' behind all issues for quick issue resolution and model improvement.
- Dashboards: Increase business alignment and confidence in decision-making by connecting ML and LLM metrics to business KPIs in a unified view
- Charts: Build customizable reports with the reports with the insights you need to gain deep understanding of your models and their impact on business outcomes
- Root Cause Analysis: Pinpoint problematic areas causing models to underperform
- Slice and Explain: Drill down on slices of data to perform exploratory or targeted analysis
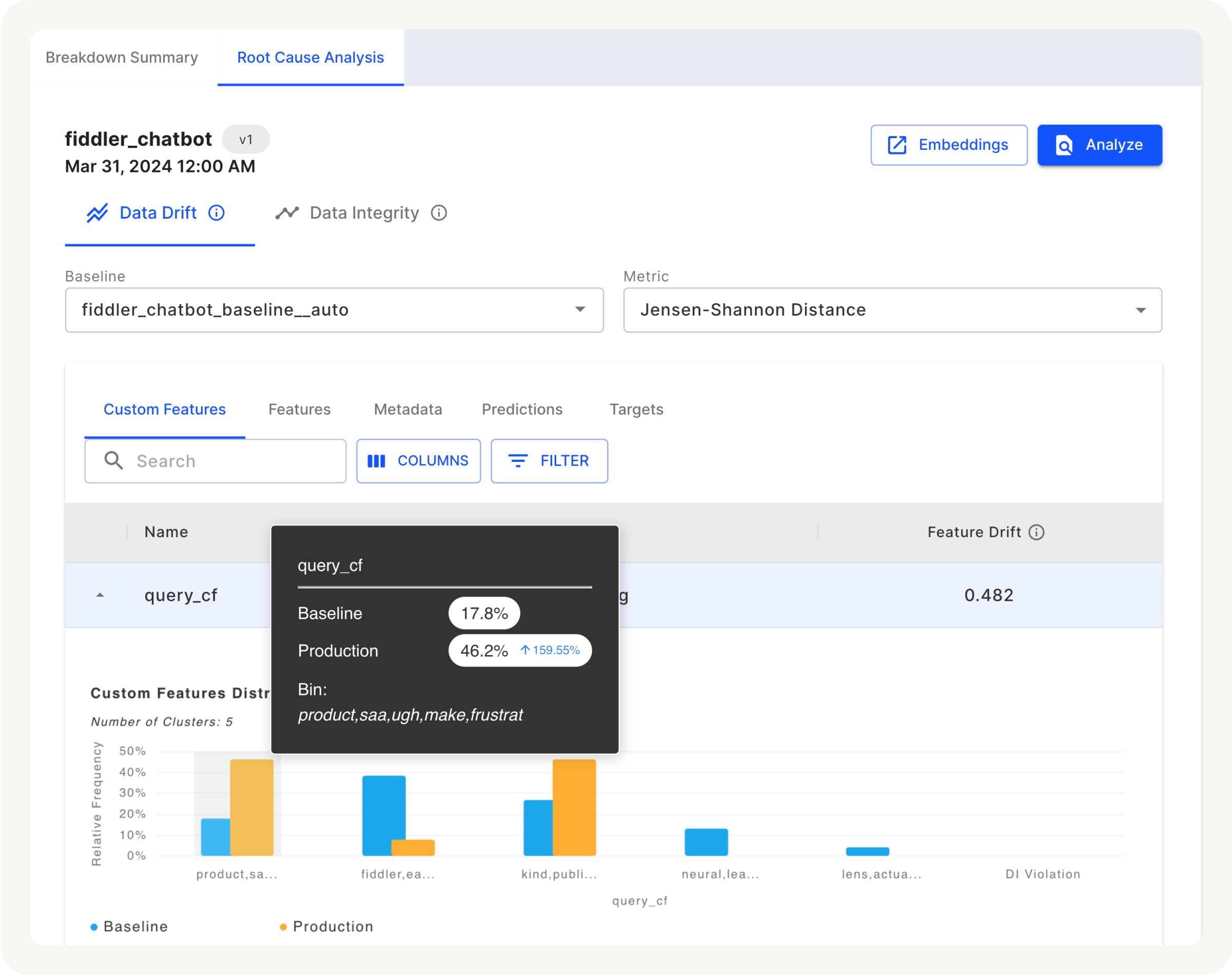
Security
Safeguard AI applications with enterprise-grade security and low-latency guardrails. Fiddler enables secure deployment in SaaS and VPC environments, preventing data leaks, prompt injection attacks, and jailbreak attempts while upholding trust and safety.
Proactively protect against security risks:
- Prevent security threats like prompt injection and adversarial attacks.
- Reduce risk exposure with compliance-driven monitoring and alerts.
- Ensure data privacy with secure deployments in SaaS and VPC environments.
