AI Observability Platform
Reduce Costs with Trusted AI Solutions
Build Your Framework for Responsible AI
Predictive models, generative AI models, and AI applications present complex operational challenges, and teams often lack enterprise-grade MLOps and LLMOps tools for model explainability or model bias assessment. As a result, too much time is spent troubleshooting, making it difficult to maintain strong performance.
Built with enterprise-scale, security, and support included, Fiddler simplifies model monitoring with a unified management platform, centralized controls, and actionable insights.
- Deliver a monitoring framework for responsible AI practices with visibility into model behavior and predictions.
- Provide deep actionable insights using explanations and root cause analysis.
- Give immediate visibility into performance issues to avoid negative business impact.
Any Model, Any Dataset
Fiddler’s out-of-the-box integrations are easily pluggable with existing data and AI infrastructure making it flexible to use.



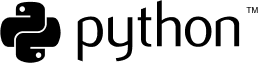


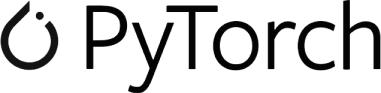




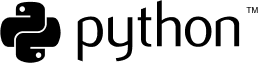


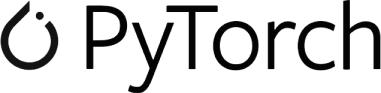




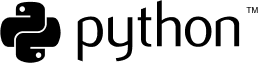


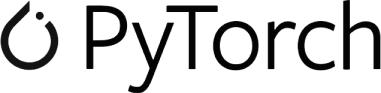


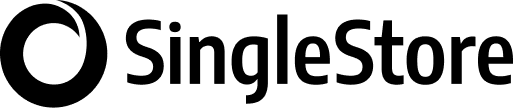

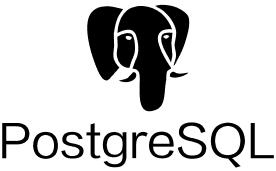


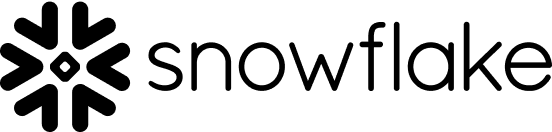


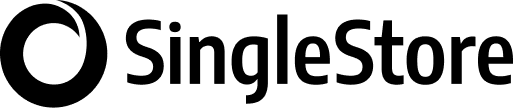

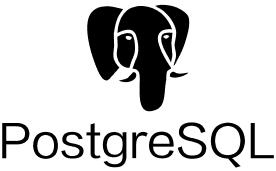


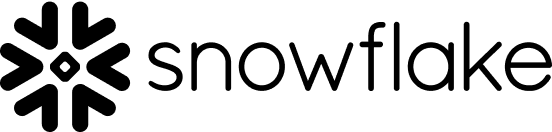


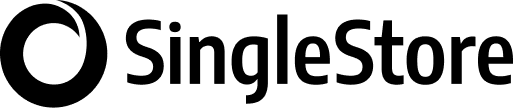

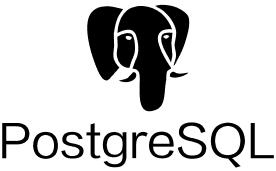


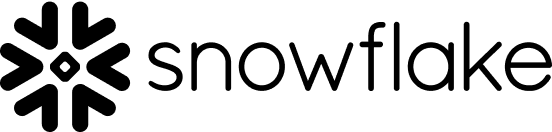


Achieve Faster Time-to-market at Scale
Do you rely on manual processes to track the performance and issues of models in training and production? Is it time-consuming and difficult to identify and attribute root causes in order to resolve issues? Do you struggle with siloed LLM and ML model monitoring tools and processes that prevent collaboration across teams?
With Fiddler, you can:
- Monitor and validate tabular and unstructured ML models faster prior to launch.
- Solve challenges such as data drift and outliers using explainable AI.
- Get real-time alerts when model performance metrics drop for an immediate fix.
- Connect model performance and predictions to business KPIs.
- Empower multiple teams to fix issues with a unified dashboard and shareable views.
Gain Confidence in Model Performance
How do you extract causal drivers in data, ML models in a meaningful way? If you don’t know or understand how a model is behaving, can you be confident in its predictions?
With Fiddler, you can:
- Provide explanations for all model predictions, delivering explainable AI at scale with easy-to-use interfaces.
- Solve challengeSupply the best explainability methods available, including Shapley Values and Integrated Gradients.s such as data drift and outliers using explainable AI.
- Bring your own explainers for faithful explanations.
- Query and replay past incidents for enhanced debugging.
- Understand model behavior with local and global explanations for multi-modal, tabular, text, and computer vision inputs.
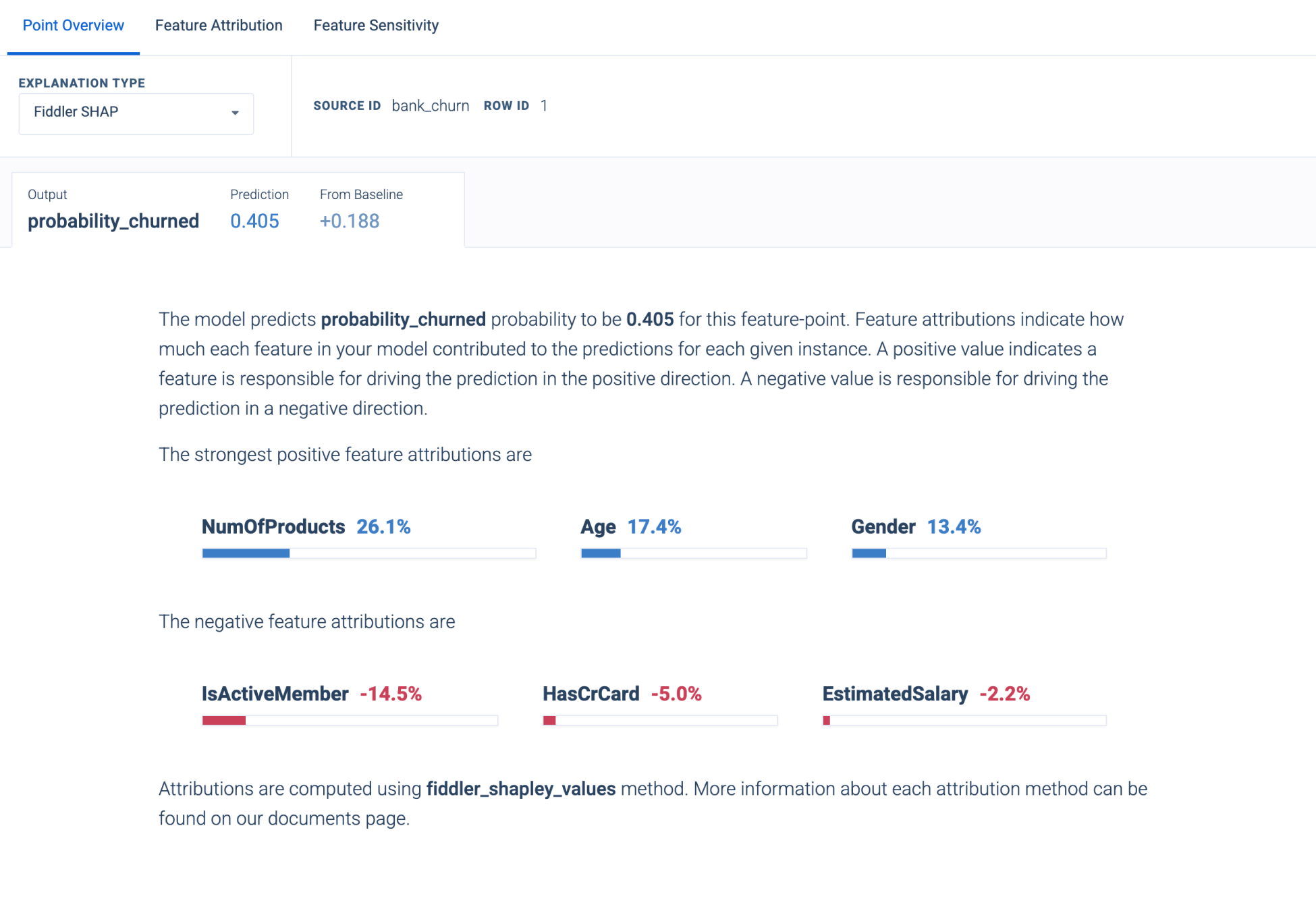
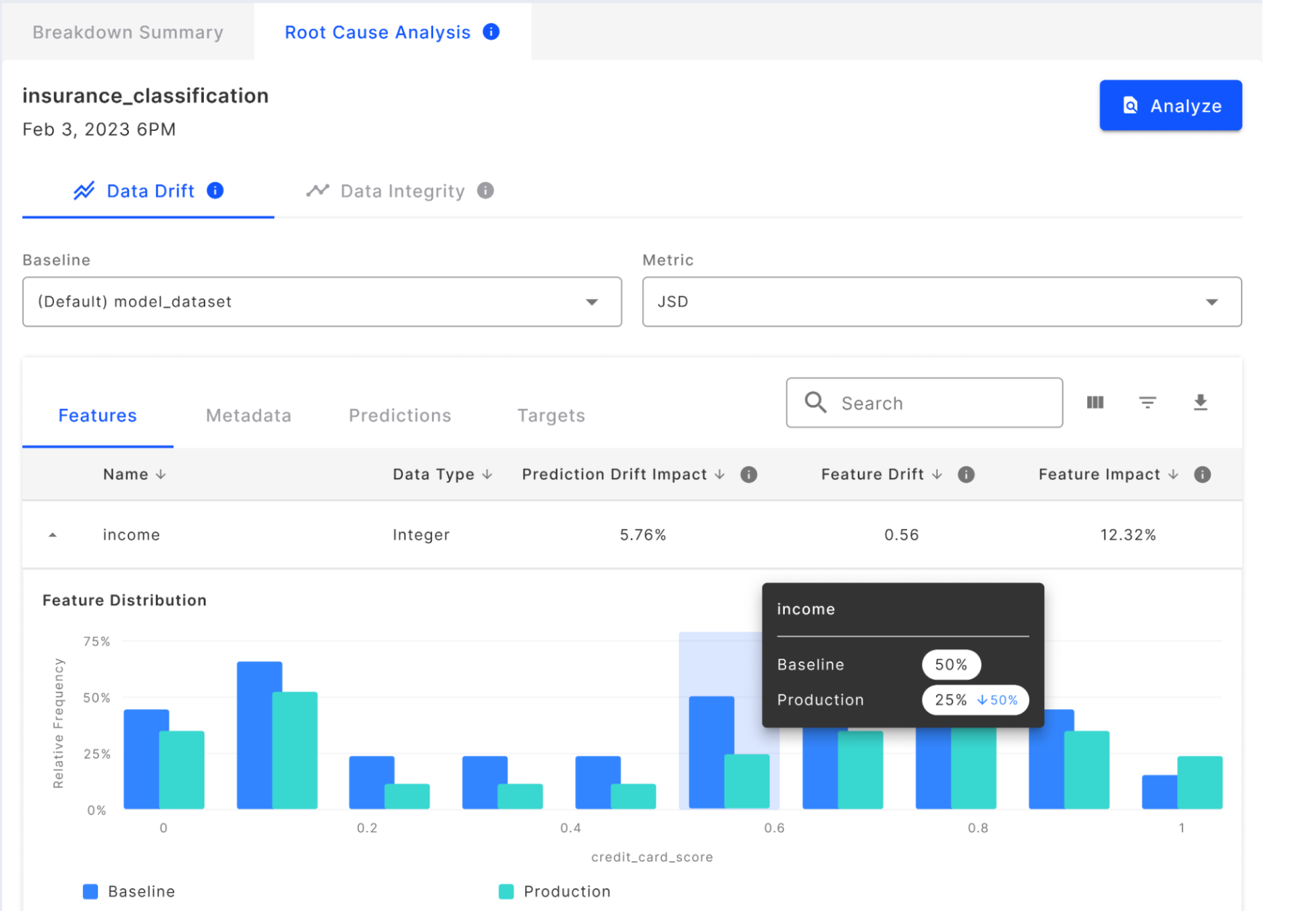
Pinpoint Root Causes and Gain Actionable Insights
What if you could understand the business impact of ineffective models? Too often, enterprise tools lack complete functionality to support ML model and LLM validation; let alone understand how to improve operational stability and make performance improvements.
With Fiddler, you can:
- Drill-down to understand where a model is failing and how to improve it.
- Test new theories using what-if analysis with predictions on altered inputs.
- Mitigate risk with access to past, present, and future model outcomes.
- Understand model behavior with local and global explanations for multi-modal, tabular, text, and computer vision inputs.
Achieve Visibility to Ensure Transparency
Lack of guidelines around operational ML and fairness make it challenging to deliver responsible AI. To ensure the exclusion of deep-rooted biases, you need explanations for ML model predictions and the ability to include humans in the decision-making process.
With Fiddler, you can:
- Make every model explainable in human-understandable terms.
- Detect and evaluate potential bias issues within training and live production datasets.
- Access standard intersectional fairness metrics such as disparate impact, equal opportunity, and demographic parity.
- Select multiple protected attributes to detect hidden intersectional unfairness across protected classes.
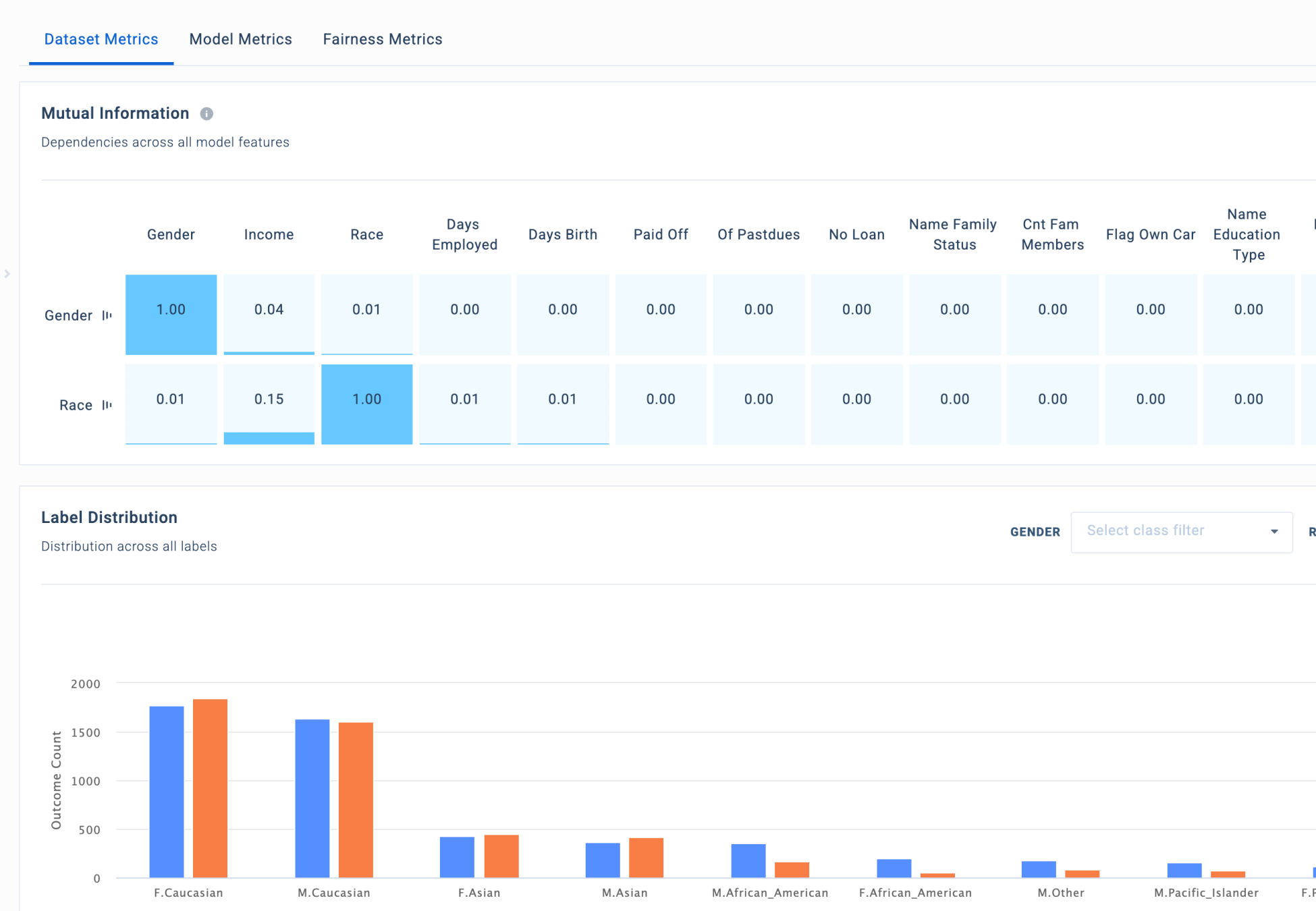
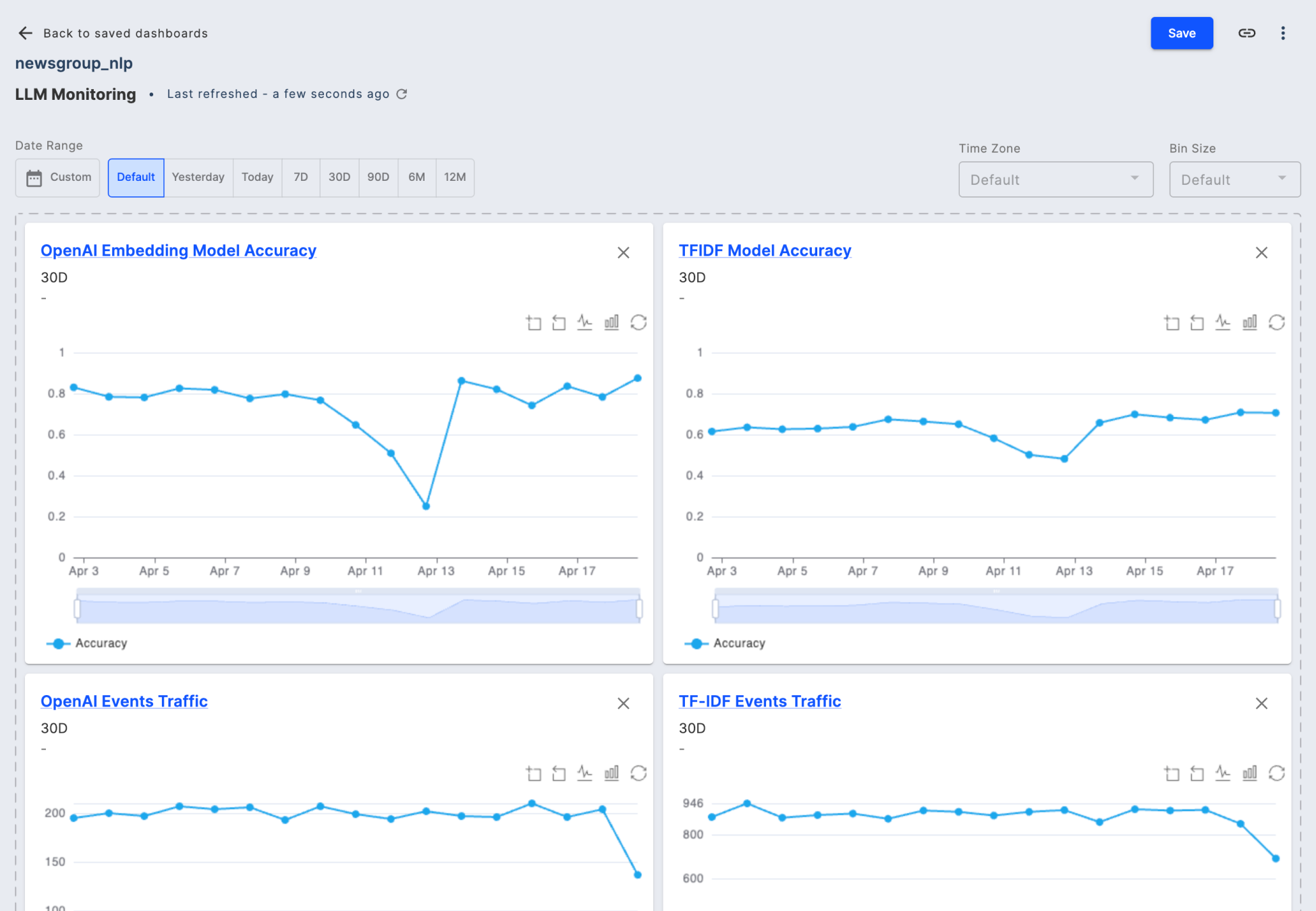
Dedicate Your Efforts to Innovation, Not In-house Systems
Are you entrenched in that age-old debate?
One thing is for certain: in-house systems are expensive to build and costly to maintain. Plus, it’s rare that an in-house system can match the breadth and depth of a purpose-built SaaS solution. Isn’t it better to gain a trusted partner for creating responsible AI?
- Operationalize the MLOps lifecycle to ensure the creation of responsible AI.
- Reduce the TCO of a homegrown solution, estimated at $750K over three years.
- Deploy a MLOps solution designed for enterprise scale with built-in security and support.
Frequently Asked Questions
What is AI observability?
AI observability is the practice of monitoring, analyzing, and troubleshooting AI models and systems in real time to ensure performance and reliability. It involves tracking data inputs, model behavior, and outputs to detect anomalies and optimize performance.
Why is AI observability important?
AI observability is crucial for detecting performance degradation, mitigating biases, and ensuring transparency. It gives businesses the confidence to deploy more AI models and applications, while maintaining trust and compliance.
What are the key features to look for in an AI Observability platform?
An AI observability platform should include key features such as metric tracking and visualization, data segmentation, bias detection, root cause analysis, and alerting systems.. These capabilities help AI models operate as expected, maintain transparency, and align with business goals while proactively identifying risks.
How can AI observability improve model performance and reliability?
By continuously monitoring model behavior, AI observability identifies errors, biases, and drift early. This allows teams to identify areas of improvement quicjkly and make adjustments, improving model or application accuracy and reliability.
What are the best methods for measuring AI impact on business performance?
AI impact can be measured using AI Observability by tracking key metrics such as revenue growth, cost savings, customer retention, operational efficiency, or custom metrics specific to your industry or business.