Customers
You’re in good company. Data science and ML teams across industries build responsible AI solutions with Fiddler.
Integral Ad Science Scales Transparent and Compliant AI Products with AI Observability
With Fiddler, IAS has experienced significant benefits:
- Reduction in monitoring operation costs due to increased efficiency
- Proactive regulatory compliance and easier demonstration of responsible AI practices to stakeholders
- A unified, standardized view of rich model insights and metrics for improved team collaboration, and audits
Read case study
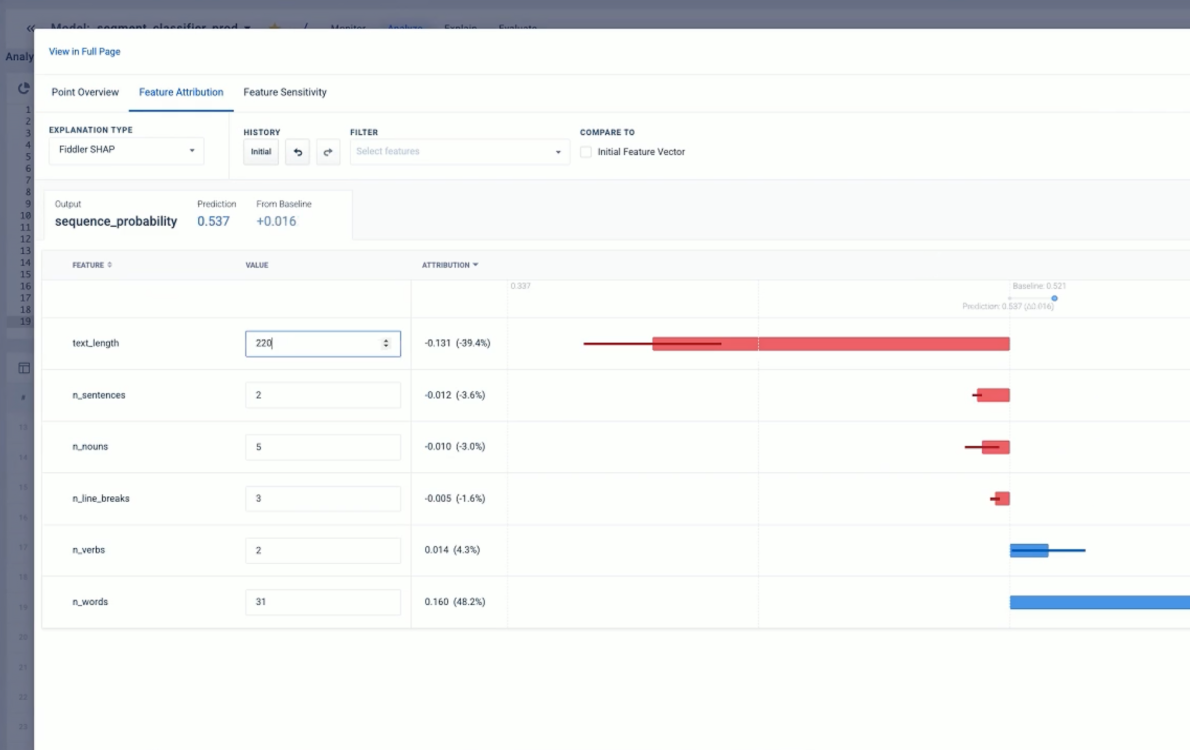
Fiddler’s robust AI Observability platform is a powerful, yet flexible addition to our MLOps framework. Since using Fiddler, we have saved one day worth of troubleshooting for every issue that Fiddler detects. Fiddler has taken full care of our monitoring needs in the background, while the data science team is focusing on what matters in the foreground.
Suryanarayana Ambatipudi
Head of Data Science, Tide
“One of the things that was appealing to IAS about Fiddler was its ability to customize the monitoring to specific model type, data volume and desired insights. Additionally, the dashboard views, automated alerting and ability to generate audit evidence also factored into the decision to work with Fiddler.”
Kevin Alvero
Chief Compliance Officer, IAS