Large language models (LLMs) are at the forefront of innovation, driving advancements in AI that offer promising benefits to enterprises. However, the deployment of these powerful models is not without its challenges. Enterprises embarking on this journey face multiple concerns, from LLM deployment and performance to ensuring correctness (hallucinations), safety (toxicity), privacy (PII), and AI compliance.
Enterprise Concerns in LLM Deployments
Enterprise leaders are eager to harness the power of LLMs to open up new revenue streams, streamline business operations, and boost customer experiences. LLMs, however, can pose risks and harm to both enterprises and end-users if they are not deployed responsibly. Enterprises risk incurring substantial fines and suffering reputational damage if, for example, their LLM-based chatbot produces toxic responses that could harm an end-user’s wellbeing, or if an end-user manipulates the chatbot into leaking private information.
Whether enterprises deploy LLMs by calling APIs, via RAG, fine-tuning, or even training their own models, they have the following AI concerns:

The New MOOD Stack for LLMOps
It is imperative for enterprises to standardize their LLMOps by using the new MOOD stack — a framework that encompasses Modeling, AI Observability, Orchestration and Data layers — with AI Observability orchestrating all the layers together, capturing the full value of LLMs.
For enterprises to obtain the full potential of LLMs, it is imperative to standardize their LLMOps using the MOOD stack — a comprehensive framework comprising Modeling, AI Observability, Orchestration, and Data layers. AI Observability is instrumental within this stack, orchestrating and enhancing the other layers by offering governance, interpretability, and LLM monitoring to improve operational performance and mitigate risks.
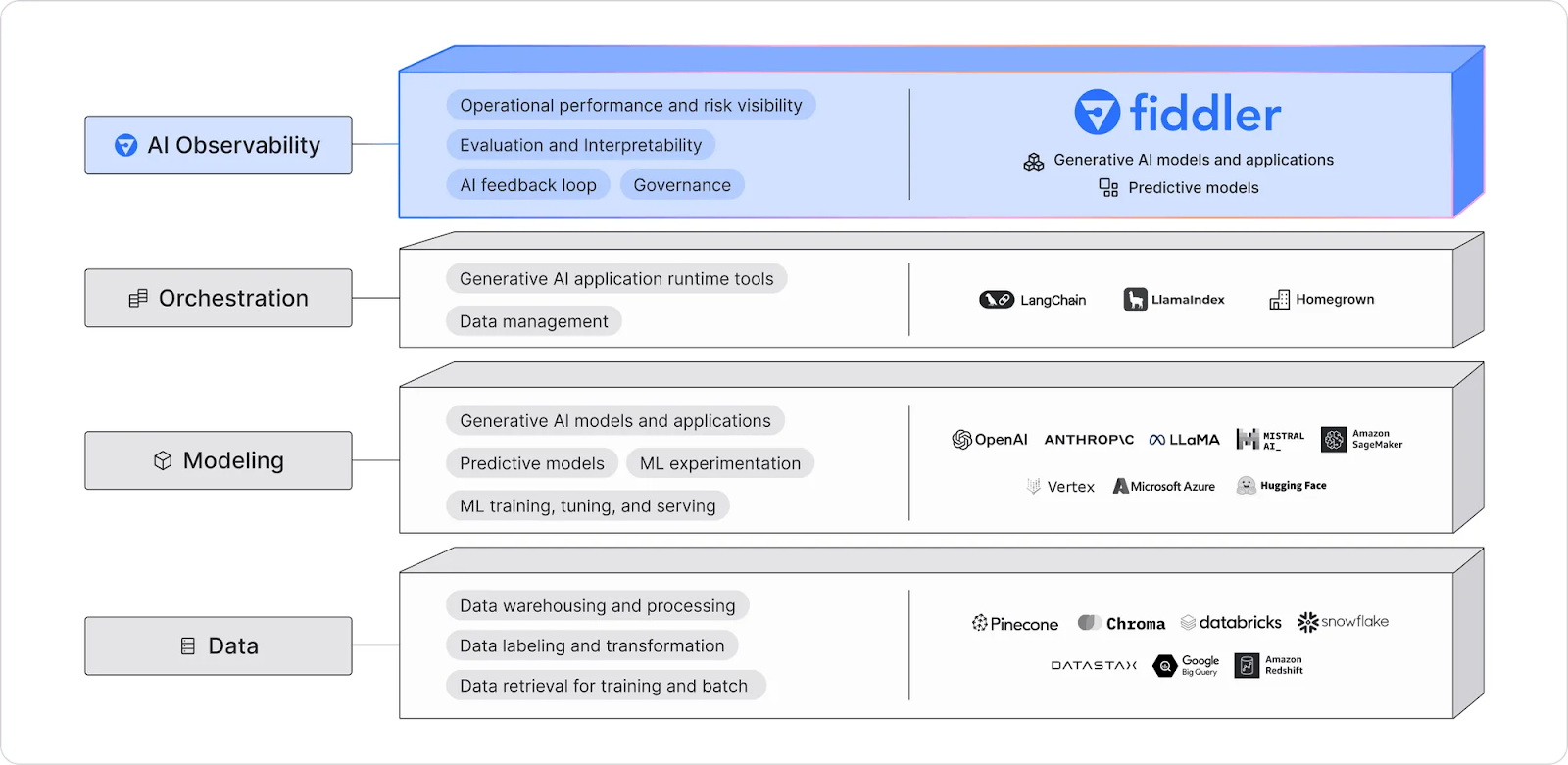
The Critical Role of AI Observability in LLM Monitoring
LLM deployments are deemed successful when they operate as intended. But how do enterprises know whether their LLM applications are doing well?
Monitoring LLM metrics is essential to ensure LLM-based applications are high performing, high quality, private, safe, correct and helpful. A comprehensive AI Observability platform not only evaluates the robustness, safety, and correctness of LLMs and prompts to boost confidence upon deployment but also continuously monitors production LLMs across various metrics. These include hallucination scores (such as answer and context relevance, groundedness, and consistency), safety metrics (including toxicity, profanity, and sentiment), privacy (specifically PII), and more.
By monitoring LLM metrics, enterprises can minimize the risks and concerns associated with LLMs, enabling them to fully leverage the benefits LLM-based applications offer to their businesses.
Read The Ultimate Guide to LLM Monitoring to learn more about the approaches of monitoring LLM metrics for successful LLM deployments.