I had the pleasure to sit down with Patrick Hall from BNH.AI, where he advises clients on AI risk and researches model risk management for NIST's AI Risk Management Framework, as well as teaching data ethics and machine learning at The George Washington School of Business. Patrick co-founded BNH and led H2O.ai's efforts in responsible AI. I had a ton of questions for Patrick and he had more than one spicy take. If you don’t have time to listen to the full interview (but I’m biased and recommend that you do), here are a few takeaways from our discussion.
TAKEAWAY 1
To make AI systems responsible, start with the NIST Risk Management framework, provide explanations and recourse to the end user to appeal wrong decisions, and establish model governance structures that are separate from the technology side of their organization.
Patrick recommends referring to the NIST AI Risk Management framework, which was released in January 2023. There are many responsible AI or trustworthy AI checklists available online, but the most reliable ones tend to come from organizations like NIST and ISO (International Standards Organization). Both NIST and ISO develop different standards, but they work together to ensure alignment.
ISO provides extensive checklists for validating machine learning models and ensuring neural network reliability, while NIST focuses more on research and synthesizes that research into guidance. NIST has recently published a comprehensive AI risk management framework, version one, which is one of the best global resources available.
Now, on the topic of accountability, there are a few key elements. One direct approach involves explainable AI, which allows for actionable recourse. By explaining how a decision was made and offering a process for appealing that decision, AI systems can become more accountable. This is the most crucial aspect of AI accountability, in my opinion.
Another aspect involves internal governance structures, like appointing a chief model risk officer who is solely responsible for AI risk and model governance. This person should be well-compensated, have a large staff and budget, and be independent of the technology organization. Ideally, they would report to the board risk committee and be hired and fired by the board, not the CEO or CTO.
TAKEAWAY 2
Individuals like Elon Musk and major AI companies may sign letters warning of AGI for strategic reasons. These conversations distract from the real and addressable problems with AI.
Patrick shared his opinion that Tesla needs the government and consumers to be confused about the current state of self-driving AI, which is not as good as it's often portrayed. OpenAI wants their competitors to slow down, as many of them have more funding and personnel. He believes this is the primary reason behind these companies signing such letters.
These discussions create noise and divert AI risk management resources toward imagined future catastrophic risks, like the Terminator scenario. Such concerns are considered fake and, in the worst cases, deceitful. Patrick emphasized that we are not close to any Skynet or Terminator scenario, and the intentions of those who advocate for shifting resources in that direction should be questioned.
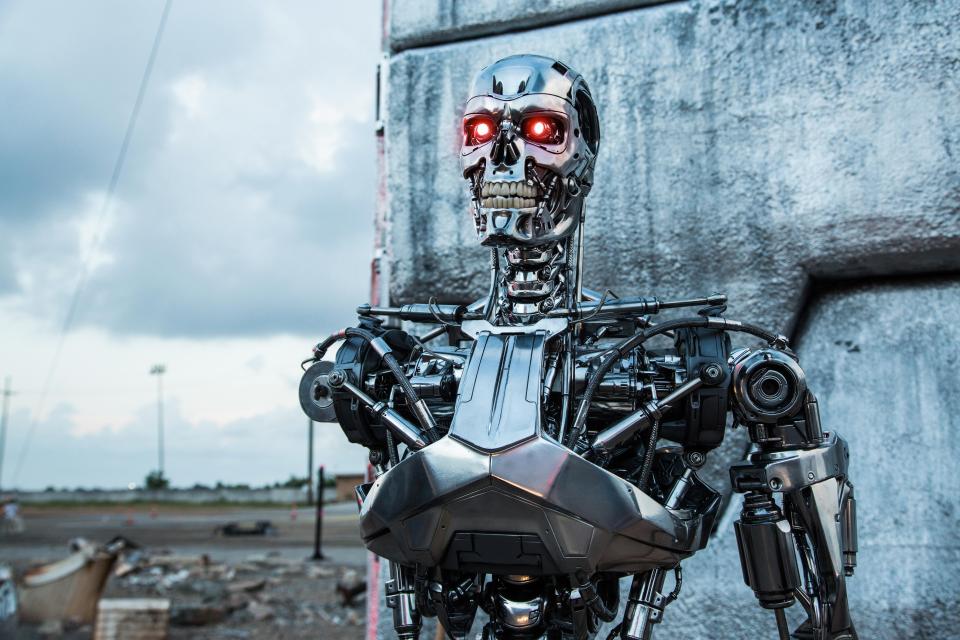
TAKEAWAY 3
Using hard metrics like the AI indecent database to motivate the adoption of responsible AI practices is better than using concepts like “fairness”.
Large organizations and individuals often struggle with concepts like AI fairness, privacy, and transparency, as everyone has different expectations regarding these principles. To avoid these challenging issues, incidents — undebatable, negative events that cost money or harm people — can be a useful focus for promoting responsible AI adoption.
The AI incident database is an interactive, searchable index containing thousands of public reports on more than 500 known AI and machine learning system failures. The database serves two main purposes: information sharing to prevent repeated incidents and raising public awareness of these failures, possibly discouraging high-risk AI deployments.
A prime example of mishandling AI risk is the chatbot released in South Korea in 2021, which started making denigrating comments about various groups of people and had to be shut down. This incident closely mirrored Microsoft's high-profile Tay chatbot failure. The repetition of such well-known AI incidents indicates the lack of maturity in AI system design. The AI incident database aims to share information about these failed designs to prevent organizations from repeating past mistakes.
Patrick had many more takeaways and tidbits to help you manage your AI risk and align with upcoming AI regulations. To learn more watch the entire webinar on-demand!