Artificial Intelligence (AI) has rapidly evolved from a promising innovation to a foundational technology driving enterprise growth. From personalized recommendations to automated decision-making, AI now powers many mission-critical systems. But with this growth comes heightened risk.
AI systems process massive volumes of sensitive data, generate impactful responses, and rely on complex third-party ecosystems. A single vulnerability can open the door to breaches, manipulation, or compliance failures. That's why AI security is no longer optional — it's essential for any enterprise adopting AI at scale.
This blog will explore why AI security is critical for modern enterprises, the risks involved, and how the Fiddler AI Observability and Security platform helps organizations confidently secure their LLM models and applications — and implement enterprise AI with security at the core.
What is AI Security?
AI security protects AI systems, models, and the data they rely on from malicious threats, unauthorized access, and misuse. As organizations increasingly adopt AI solutions to transform operations, security measures must evolve parallel to protect their integrity and ensure responsible deployment.
Key Components of AI Security
To effectively protect AI systems, enterprises must address several foundational pillars of security, supported by robust AI tools designed for monitoring, analytics, protection, and control:
- Access Control: Only authorized personnel can interact with AI models or training data.
- Data Integrity: Preventing data poisoning or tampering that can skew model behavior.
- Model Protection: Safeguarding against reverse engineering, theft, or malicious manipulation.
- Monitoring and Analytics: Observing LLM prompts and responses in real-time and understanding model outputs to detect hallucinations, safety violations, or prompt injections.
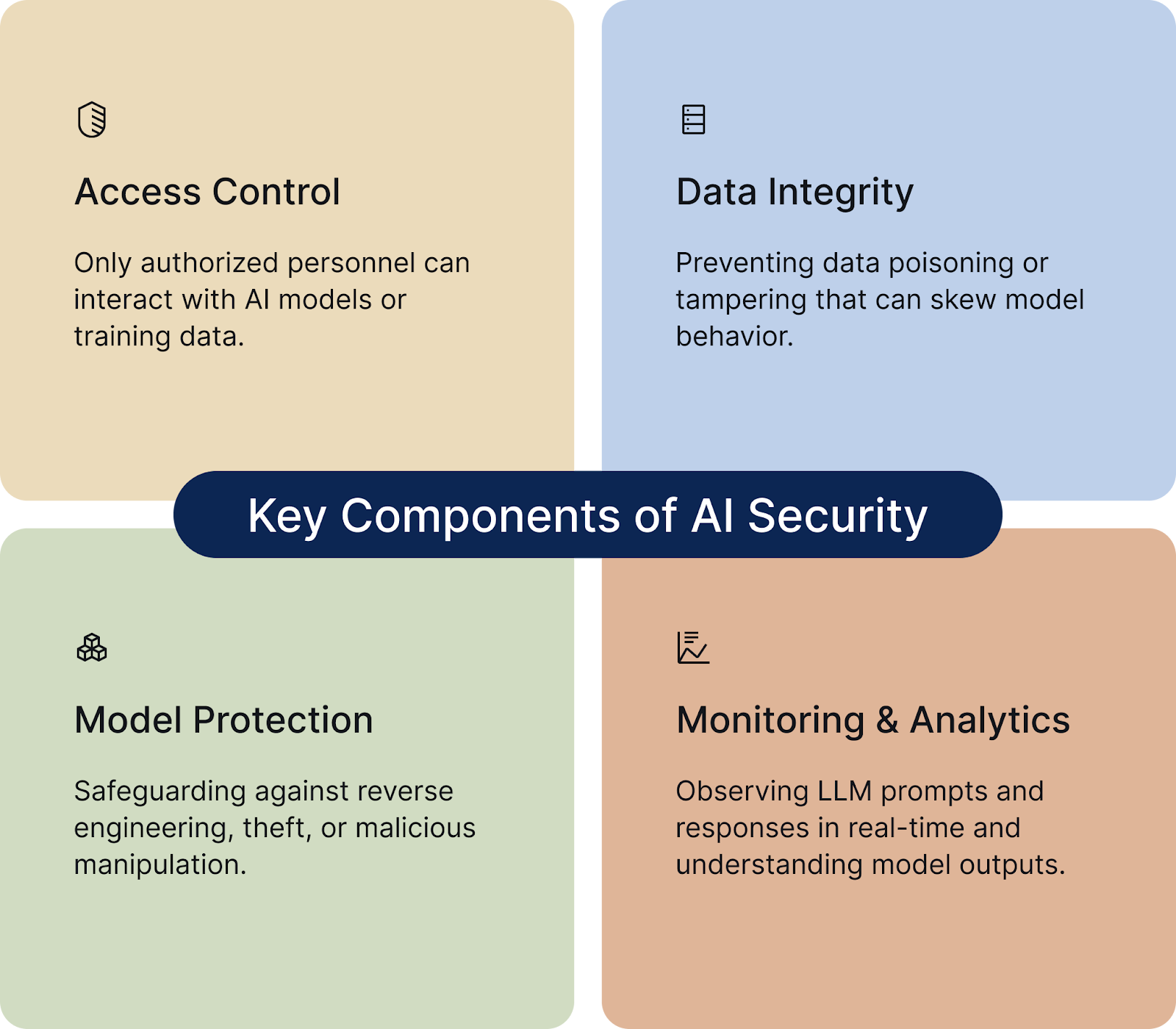
As organizations use AI for customer service, financial advisory, hiring, healthcare, and beyond, any security gap can lead to severe consequences. Investing in enterprise AI security builds resilience, ensures compliance, and protects user trust and business value.
AI Security Risks for Enterprises
AI technologies are uniquely vulnerable to a range of attacks. Their complexity, reliance on vast datasets, and predictive nature make them prime targets for malicious actors looking to exploit weaknesses at any stage.
Organizations must understand and address risks across three critical areas, data, models, and the supply chain, to effectively secure enterprise AI.
1. Data Vulnerabilities
Enterprise AI systems depend on vast datasets to train and operate. These datasets often contain sensitive, regulated, or proprietary information, making them a prime target for security vulnerabilities.
Key Risks:
- Data Breaches: Unauthorized access to training or inference data.
- Data Poisoning: Injection of corrupted data to manipulate model response.
- Adversarial Attacks: Malicious prompts that manipulate a model to give out private information.
These risks can lead to GDPR or CCPA violations, triggering costly fines, brand damage, and lost customer trust if not addressed. Securing AI data is the first line of defense for businesses.
2. Model Exploits
Beyond data, models — including generative AI systems — are significantly vulnerable to exploitation as AI adoption accelerates across industries.
Common threats include:
- Model Inversion: Reconstructing sensitive training data by analyzing model outputs.
- Model Theft: Cloning or replicating proprietary models through exposed APIs or reverse engineering.
- Hallucinations: Producing inaccurate outputs due to unsecured or unmonitored models.
These threats can lead to data leakage, regulatory violations, and serious business consequences without robust AI model security. As AI adoption grows, securing models becomes just as critical as securing the data they rely on.
3. Supply Chain Threats
Many enterprises use third-party APIs, pre-trained models, and open-source libraries to accelerate development. But each component introduces potential backdoors.
Key concerns:
- Insecure Dependencies: Vulnerabilities in third-party code.
- Model Supply Chain Attacks: Manipulating or compromising foundational models.
- Data Mishandling by Vendors: Violating internal or external data security policies.
Without robust AI security standards, enterprises risk inheriting these vulnerabilities — and passing them on to customers.
How AI Security Helps Protect Enterprise Models
Effective AI for enterprises requires more than traditional cybersecurity. It demands purpose-built security controls, standards, and real-time monitoring customized to AI's unique needs. This approach includes securing data, models, and the infrastructure where models operate, making endpoint security a critical layer of defense in any enterprise AI strategy.
Data Protection
A secure AI system starts with safeguarding the data it consumes, generates, and shares — especially as enterprises expand data access across teams and platforms.
Key practices include:
- End-to-End Encryption: Ensures sensitive data is protected at rest and in transit.
- Access Control: Role-based permissions limit who can view or modify data.
- Real-Time Detection: Automated tools surface unusual patterns, breaches, or anomalies, which is crucial when automating routine tasks at scale.
Threat Detection and Response
Threats to AI models evolve fast, so security needs to be agile.
AI security measures include:
- Threat Hunting: Using AI to detect AI-specific risks like prompt injection or drift.
- Trend Analytics: Spotting deviations in model outputs that signal manipulation.
- Automated Remediation: Isolating and fixing issues without human intervention.

Scaling AI Security at the Enterprise Level with Fiddler
Securing AI models isn't easy, especially at the enterprise scale. That's where the Fiddler AI Observability and Security platform steps in.
1. Analytics-Driven Risk Mitigation
Fiddler uses advanced analytics to perform root cause analysis to pinpoint and contextualize risks in real time.
Fiddler Features that Drive Enterprise AI Security:
- Guardrails: Define safety boundaries, usage policies, and ethical constraints to limit unsafe or unintended outputs. Fiddler's AI Guardrails help protect LLM applications from security risks, prompt injections, and harmful content generation.
- Model Monitoring: Continuously observe user prompts, model responses, and usage patterns in real time. This capability enables early identification of drift, hallucination, toxicity, jailbreak attempts — supporting enhanced threat detection and reducing the likelihood of unnoticed vulnerabilities or unsafe LLM responses.
- Trust Assessments: Evaluate if AI models make reliable, aligned decisions. Fiddler helps enterprises assess their models — building trust through high-quality, secure, and safe LLM applications that reflect business values and meet regulatory standards.

These features empower teams to respond confidently and quickly to issues before they become full-blown threats.
2. Strengthening Data Security
Fiddler enhances AI data security by combining visibility and protection.
How Fiddler helps:
- Automatically monitors for data anomalies, misuse, or unauthorized access.
- Protects model outputs with Guardrails to prevent unsafe or unintended responses.
- Manages role-based access controls for authorized personnel, and all data reside within the customer’s cloud or VPC environment.
3. Securing AI Models
LLMs and other AI models are only as secure as the systems supporting them. Fiddler offers robust AI model security capabilities.
These capabilities include:
- Model access management and version control.
- Real-time drift detection to spot unusual usage or performance changes.
- Logging and audit trails to ensure accountability.
4. Managing Supply Chain Risk
Fiddler recognizes the growing need to secure the AI supply chain — from foundational model providers to third-party services.
Fiddler's approach:
- Continuously monitors third-party components.
- Flags potential LLM vulnerabilities from providers like OpenAI, Anthropic, Meta, and Google.
- Promotes secure AI post-deployment best practices.
With Fiddler, enterprises gain the control they need to navigate a rapidly evolving AI ecosystem.
Securing the Future: Why Enterprise AI Needs Protection Now
As AI becomes foundational to enterprise business operations, organizations must proactively address the risks it introduces. From AI data security to model protection, the threat landscape continues to expand and evolve rapidly.
AI will continue to play a pivotal role in driving innovation, operational efficiency, and customer engagement. That's why AI security should be treated as a long-term strategic priority — not an afterthought — and guided by robust security frameworks tailored to the complexities of AI technologies.
Fortunately, enterprises don't have to navigate these challenges alone. Ready to protect your enterprise AI applications? Learn how Fiddler AI Security enables organizations to deploy and manage AI models confidently and take their AI strategy to the next level.
Frequently Asked Questions about AI Security
1. Why is security important in AI?
AI systems handle sensitive data and make critical decisions. Without proper security, they're vulnerable to attacks, data breaches, and manipulation — putting users, businesses, and reputations at risk.
2. What is the use of AI for enterprises?
Enterprises use AI to streamline operations, automate workflows, personalize customer experiences, and make data-driven decisions. It drives efficiency, innovation, and competitive advantage.
3. Is AI a threat to security?
AI can be both a tool and a target. While it enhances cybersecurity through automation and threat detection, poorly secured AI systems can introduce new risks, such as data leaks, adversarial attacks, or model misuse.
4. What is responsible AI for enterprises?
Responsible AI is an AI system's ethical, transparent, and secure development and deployment. This means ensuring fairness, accountability, privacy, and regulatory compliance for enterprises while building Responsible AI with observability to monitor, explain, and improve model behavior.
5. What are the data security concerns with AI?
AI often processes large volumes of personal or proprietary data. Key concerns include data breaches, unauthorized access, data poisoning, and compliance violations — making strong data protection essential.