The Rise of MLOps Monitoring
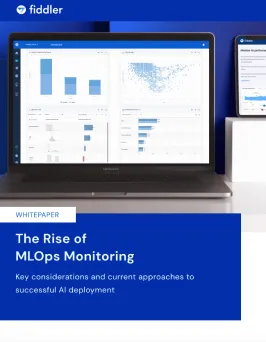
Successful AI deployments require continuous ML monitoring to prove business value on an ongoing basis. Whether solving for data drift or model bias, retraining models, surfacing live performance metrics, or gaining visibility into the black-box of ML models, ML monitoring is an essential piece of the MLOps lifecycle - and often the most difficult part. How will you rise to the challenge?
This paper explores:
- The evolution of ML Monitoring
- The 7 key challenges for MLOps
- How ML teams can solve these challenges