In the previous post on Fiddler’s 4th Explainable AI (XAI) Summit, we covered the keynote presentation and its emphasis on the importance of directly incorporating AI ethics into a business.
In this article, we shift the focus to banking, an industry that is increasingly using artificial intelligence to improve business outcomes, while also dealing with strict regulation and increased public scrutiny. We invited technical leaders from several North American banks for a panel discussion on best practices, new challenges, and other insights on Responsible AI in finance. Here, we highlight some of the biggest themes from the conversation.
Watch the full recording of the Responsible AI in banking panel.
AI Applications in Banking
Many banking functions that were once entirely manual are now partly or even fully automated by AI. AI helps define the who, what, when, and how of banks’ marketing offers for opening new savings accounts or credit cards. AI performs fraud detection, keeping the entire financial system more secure and reliable. AI even plays a part in some banks’ credit scoring systems and weighs in on the outcome of loan applications.
The breadth of AI use cases in finance is vast, so it’s helpful to categorize applications by model criticality: the directness of impact a model has on business decisions. If an AI model is only advising a human in making a decision, that is less critical than another model autonomously making a decision. The significance of the decision to the overall business also factors into measuring model criticality.
Model criticality affects the way an organization manages and improves its systems. As panelist Lory Nunez (Senior Data Scientist, JP Morgan Chase) explained, “Normally, the level of oversight given to our models depends on how critical the model is.” Ioannis Bakagiannis (Director of Machine Learning, Royal Bank of Canada) offered the example of sending out a credit card offer vs. declining a mortgage. The latter is a much more sensitive use case with substantially more brand risk. Thinking about models in terms of criticality is a useful framework in prioritizing efforts to promote Responsible AI.
Challenges To Address with Responsible AI
The panelists covered a number of recurring challenges in AI as applied to finance and more generally.
Algorithmic Bias
Allegations of bias in business-critical AI models have made headlines in the past. Krishna Sankar (VP & Distinguished Engineer, U.S. Bank) noted, “Even if you have the model, it is working fine, everything is good, but it does some strange things for a certain class of people. At that point you have to look at it and say, ‘No, it'll not work.’” Bias amplification can exacerbate these risks by taking small differences between classes of people in the input and exaggerating these differences in the model’s output.
Bakagiannis added, “We have certain protected variables we want to be fair and treated the same, or almost the same because every protected variable has different preferences.” It’s important to regularly monitor these properties to ensure that algorithms remain unbiased over time.
Explainability
A perennial critique of AI is that it can be a “black box.” Daniel Stahl (SVP & Model Platforms Manager, Regions Bank) explained that model transparency is valuable because data scientists, business units, and regulators can all understand how a model came up with a particular output. Regarding business units, Stahl said, “Having explanations for why they're seeing what they're seeing goes a long way to having them adopt it and have trust in that model.” On top of catering to internal stakeholders, it’s equally important to make models explainable to customers.
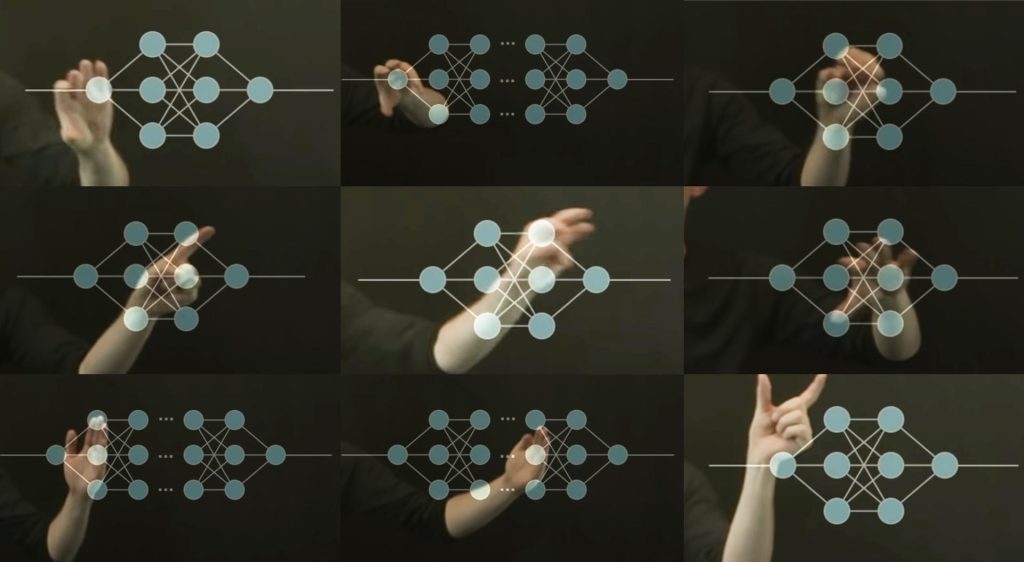
Data Quality
A model constitutes both its algorithmic architecture as well as the underlying data used for training. Even if a model is minimally biased at one point in time, shifts in the data it consumes could introduce unforeseen biases. “We have to pay attention to the non-stationarity of the world that we live in. Data change, behaviors change, people change, even the climate changes,” acknowledged Bakagiannis. Therefore, it’s a good idea to pay close attention to feature distributions and score distributions over time.
Nunez also commented on a gap in explainability: With all the focus on explaining a model’s algorithms, explanations around the data itself (such as how the data was labeled and whether there was bias) can become an afterthought. As Sankar added, “The model reflects what is in the data,” making it critical to have representative data across all classes of users the model serves.
Best Practices for Institutionalizing Responsible AI
The panelists also discussed best practices for operationalizing Responsible AI principles.
Differentiate between statistical and business significance
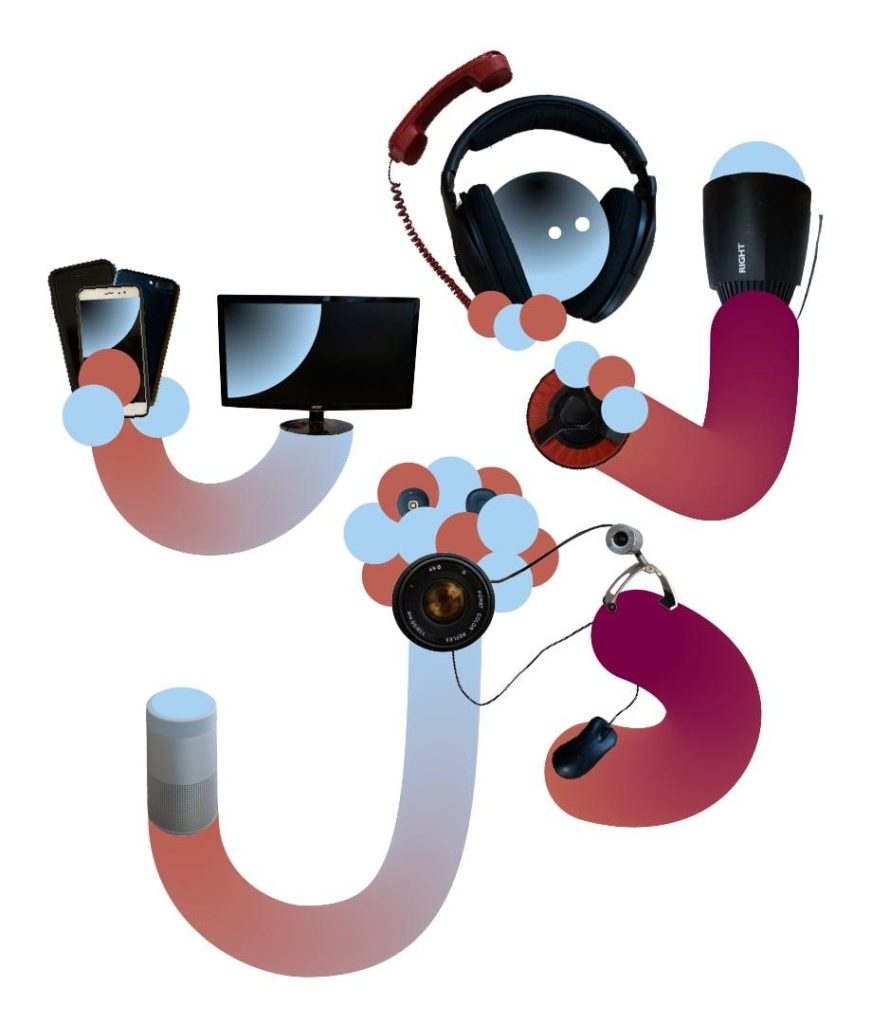
Recognizing which elements of a model are most relevant to business decisions can prevent over investment in AI for AI’s sake. “Statistical significance doesn’t mean business significance,” explained Sankar. For example, a model may have a statistically significant 0.1% improvement in targeting customers with an offer, but the magnitude of this impact may be insignificant to the business’s broader objectives.
Choose the appropriate amount of model complexity
When would you choose a complex model vs. a simpler one? As Nunez pointed out, “simple models are easier to explain.” There needs to be a good reason for choosing a complex model, such as providing a significant bump in performance. Or, as Stahl explained, a complex model may be “able to better accommodate regime changes” (changes to the data and environment).
Start small and scale models up
To overcome resistance and minimize regulatory risk, the panelists recommended using AI first as an analytical tool to assist human-made decisions, and only then scaling up to automated use cases. As part of that process, Nunez explained, organizations ought to “give [decision makers] a platform to share their feedback with your model” to ensure that the model is explainable and fair before it gets autonomy.
Measure and track improvements
With regulatory requirements in the finance industry, being able to measure progress in Responsible AI is a top priority. These measurements can be both qualitative and quantitative. Maintaining a qualitative feedback loop with users can help teams iterate on feature engineering and ensure that a model is truly explainable. On the other hand, as Sankar explained, quantitative measures like intersectional impact and counterfactual analysis can check for bias and explore how models will behave with various inputs.
Learn More
Fiddler, with its solutions for AI explainability, Model Performance Management, and MLOps, helps financial organizations and other enterprises achieve Responsible AI. Contact us to talk to a Fiddler expert!
On behalf of Fiddler, we are extremely grateful to our panelists for this productive discussion on Responsible AI in banking:
- Krishna Sankar, VP & Distinguished Engineer, U.S. Bank
- Daniel Stahl, SVP & Model Platforms Manager, Regions Bank
- Lory Nunez, Senior Data Scientist, JP Morgan Chase
- Ioannis Bakagiannis, Director of Machine Learning, Royal Bank of Canada
You can watch all the sessions from the 4th XAI Summit here.