Explainability is the most effective means of ensuring AI solutions are transparent, accountable, responsible, fair, and ethical across use cases and industries. When you know why your models are behaving a certain way, you can improve them — and share your knowledge to empower your entire organization. As part of Fiddler’s 3rd Annual Explainable AI summit, we brought together a group of experts in research, finance, health care, and the tech industry. We asked our panelists to share some insights from their specific domains, regarding how Explainable AI is currently being used, what some of the challenges are, and some exciting areas for future development. We’ve distilled the key points in this article, but you can also watch the full panel discussion here.
Current use cases
As Sarah Hooker (Research Scholar, Google Brain) described, early research in interpretability was mostly focused on explaining a single prediction. Now, Hooker is excited about “equipping practitioners with skimmable tools that allow them to navigate the model’s learned distribution.” Using tools to help model practitioners become more efficient “is becoming an ever more urgent challenge because of the scale of our datasets, which are ballooning in size.”
Amirata Ghorbani (PhD Candidate in AI, Stanford University) noted that in healthcare research, Explainable AI was once a nice-to-have — but not anymore. Doctors are now more involved in auditing models, and “interpretability has become another metric that you have to satisfy for your paper to be accepted.” In the finance industry, model risk management is a well-developed practice. But there is an increasing need to generate data to share with academic partners or run simulations. For Victor Storchan (Senior Machine Learning Engineer, JPMorgan Chase & Co.), “the question is, how do we make sure that the data is generated in an interpretable way?”
Pradeep Natarajan (Principal Scientist, Amazon Alexa AI) sees interpretability as the end-to-end equivalent of software testing for machine learning: from detecting errors, to understanding why they happen, to correcting them. But it’s more complex than debugging a single model. “Most production systems are sequences of multiple machine learning systems — so which system caused the error, and how did those errors accumulate as you go down the chain?”
Explainable AI in a changing environment
How can interpretability help model practitioners navigate a changing world? As Storchan explained, this is a very important question for an industry like finance, where success or failure hinges on predicting market shifts caused by external events. Regulators like FINRA look at hundreds of billions of market events per day to understand model drift. Explainable AI can help understand what the data changes are, and why they’re happening, so practitioners can build a better version of the model or develop a more robust data set.
Highly regulated industries like finance have a practice of identifying and mitigating against known risk factors — and this is an area where other AI fields like natural language processing and computer vision need to catch up, Natarajan explained. “We haven’t yet systematically defined what are the factors you should be benchmarking your algorithms against, and there is also not an established ecosystem to discover and add new factors on an ongoing basis.”
Ghorbani added that “it’s not enough anymore just to say that these are the important pixels. You want to know: Is it because there’s a wheel in the image? Is it because of the sky?” We need to explain models in terms humans can understand, because humans — and specifically domain experts, whether in finance, healthcare, or other industries — need to audit machine learning systems. “I think the most promising tools allow the domain user to control how they can explore the data set,” said Hooker.
Challenges of using Explainable AI
The main challenge of using Explainable AI right now is, as Ghorbani put it, “knowing what to do with it.” In other words, we might be able to interpret how and why a model is wrong, but we’re stuck when it comes to retraining a model that will yield better results. “There’s no closed source solution from data to the trained model,” said Ghorbani. “It’s a very difficult path to go back from — you cannot just take gradients.” Hooker agreed that “it’s a very rich, underserved research direction, to think about the training process itself.”
One of the challenges in interpretability research, according to Hooker, is that “we’ve been terrible about translating our ideas to software.” This is partly an engineering problem, and partly a scientific one. Since some of the strategies from research can be “exorbitant in cost,” productionizing these ideas means coming up with cheaper proxy methods that can get at the same result.
For Natarajan, one key challenge is identifying the risk factors that will help AI systems avoid bias, such as speech recognition that underperforms on certain accents. Ultimately, interpretability should go beyond just explaining what’s happening, and begin “providing actionable insights that developers or owners of models can act on.” In finance, there’s also a need for better and more efficient model diagnostic methods. This is especially important for addressing regulatory challenges, such as nondiscrimination requirements from GDPR.
The future of Explainable AI
Finally, we asked the panelists about the forward-thinking interpretability work that they find particularly interesting.
For Hooker, one focus is developing constraints related to what humans are capable of interpreting, and baking these constraints into models, so that the results are more meaningful. In addition to identifying factors for model evaluation, Natarajan is interested in classifying model insights and using these insights to continually improve models. For Storchan, a long-term goal is using Explainable AI to help eradicate financial crime.
Ghorbani is excited about the potential for humans to learn from ML models. “In our lab we worked on a paper where we could predict age, gender, height, weight, given someone’s current echocardiogram.” This is something that machines are better at than human doctors. Google has written a paper making similar predictions based only on a retina image. But no one has yet been able to figure out how these models made their predictions. As Ghorbani explained, this might be considered the “final test of interpretability: learning from machine learning.”
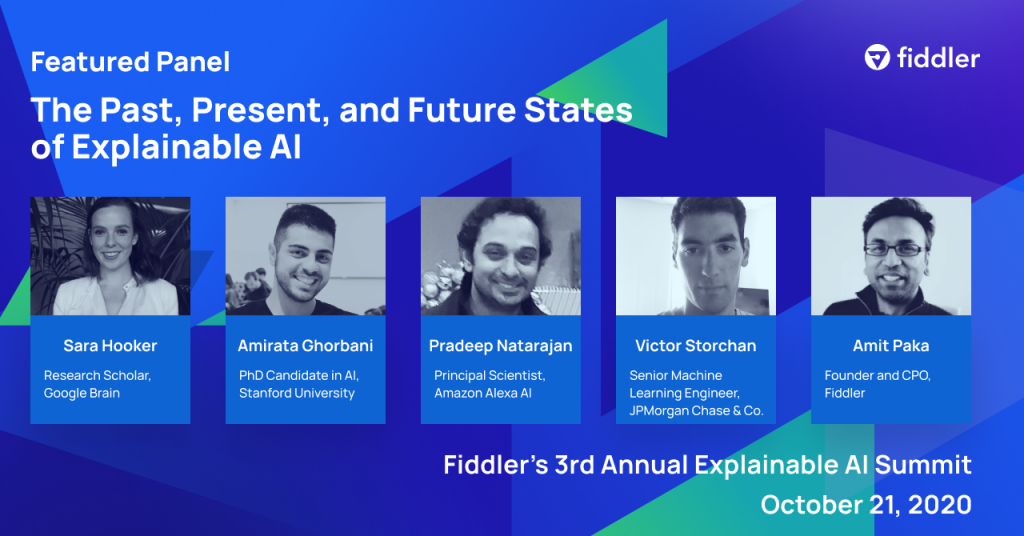
This conversation was part of Fiddler's 3rd annual Explainable AI Summit on October 21, 2020.
Panelists:
Amirata Ghorbani, PhD Candidate in AI, Stanford University
Sara Hooker, Research Scholar, Google Brain
Pradeep Natarajan, Principal Scientist, Amazon Alexa AI
Victor Storchan, Senior Machine Learning Engineer at JPMorgan Chase & Co.
Moderated by Amit Paka, Founder and CPO, Fiddler
Other Explainable AI Summit Conversations:
How Do We Build Responsible, Ethical AI?
Explainable Monitoring for Successful Impact with AI Deployments