We are thrilled to announce several major upgrades to the Fiddler Model Performance Management (MPM) platform. We’ve heard from customers asking how we can help them launch new AI initiatives and further adopt responsible AI at scale. Today, we’ve delivered product capabilities that enable ML and Data Science teams to achieve faster and better business outcomes.
Some of the biggest improvements added to the Fiddler MPM platform include giga-level scalability, natural language processing (NLP) and computer vision (CV) monitoring, a solution to better monitor class imbalance, and an intuitive user interface for seamless user experiences. With these new capabilities, customers are empowered to build more complex models to resolve advanced use cases, gain a deeper understanding of their unstructured data, such as text and images, and discover low-frequency events to further improve the accuracy of model predictions.
Giga-level scalability
ML teams are now empowered to build more complex models to achieve advanced AI use cases with Fiddler’s elevated scalability. Fiddler’s giga-level scalability supports complex models that require larger training datasets in the GBs and allows large-scale ingestion of production data.
Understanding unstructured model behavior
Customers can now realize untapped business opportunities involving complex ML use cases with unstructured data. Enterprises who have models on both structured and unstructured data can now use Fiddler to effectively monitor model drift on their production data.
By incorporating NLP and CV monitoring in their ML, companies can explore more advanced use cases such as:
- Medical practitioners can gain greater accuracy in recognizing patterns as variants of illnesses change
- Manufacturers can be alerted when the type of defects change in a defect detection CV model
- Automakers can improve passenger safety by enabling cars to detect a change in weather patterns or a change in the road environment (e.g: construction zone further ahead)
Data scientists can easily monitor high-dimensional vectors using Fiddler’s NLP and CV monitoring. This new feature also enables monitoring a group of univariate features together to detect multi-dimensional data drift. A clustering-based binning algorithm is used to create univariate histograms of multi-dimensional data.

Reveal data drift from class imbalance
ML teams can now quickly discover drift in rare classes of their imbalanced production data. Models for fraud detection, for example, need to uncover drift in the minority class since fraud events happen sporadically and are critical to detect accurately. Inability to identify unusual drift in minority classes can cost companies millions of dollars.
Class imbalance is quite common and impacts companies across industries. We have talked to many customers who see the value in identifying low-frequency, or true positive, events, such as:
- Gaming companies will be alerted when more fraudulent transactions including subtle variations occur that would otherwise cost millions of dollars in potential revenue
- Organizations can protect their advertising efforts by detecting higher-than-usual ad click rates to identify malicious behavior that impacts the bottom line
- eCommerce platforms are immediately alerted when a slight change in purchase patterns occur within a product category
Fiddler helps customers find a needle in a haystack by monitoring for class imbalance. Without making changes to the way drift is often calculated, it would be nearly impossible to pick up this substantial change in, say, fraudulent activity. This is because the normal drift calculation looks at the prediction distribution as a whole. In the grand scheme of things, there is not much change in the overall distribution due to a high class imbalance. Fiddler’s solution relies on a weighting system that allows users to either set global weights to each class, which would upweight the events from the minority class so that the resulting histogram can capture changes in prediction drift effectively, or alternatively provide event-level weights if they need more granular control of the weighting. This allows for changes in the minority class to become obvious when tracking drift. Users can confidently monitor fraud models and readily take action when rare events surface.
Learn how to monitor for class imbalance in Fiddler
Unified view from a single pane of glass
Our new centralized UI helps ML engineers and data scientists streamline their ML workflows, from high-level model performance overview to granular analytics insights for drift issues. Through a single pane of glass, ML teams have a seamless unified view to quickly track how models are performing, uncover the root cause of model drifts using powerful dashboards, and collaborate with the same shared information making it easy for them to identify and analyze the source of an issue.
ML teams can track long running async jobs, get early warnings through customizable alerts, and drill down on model information, such as drift metrics, traffic, and the number of triggered alerts.
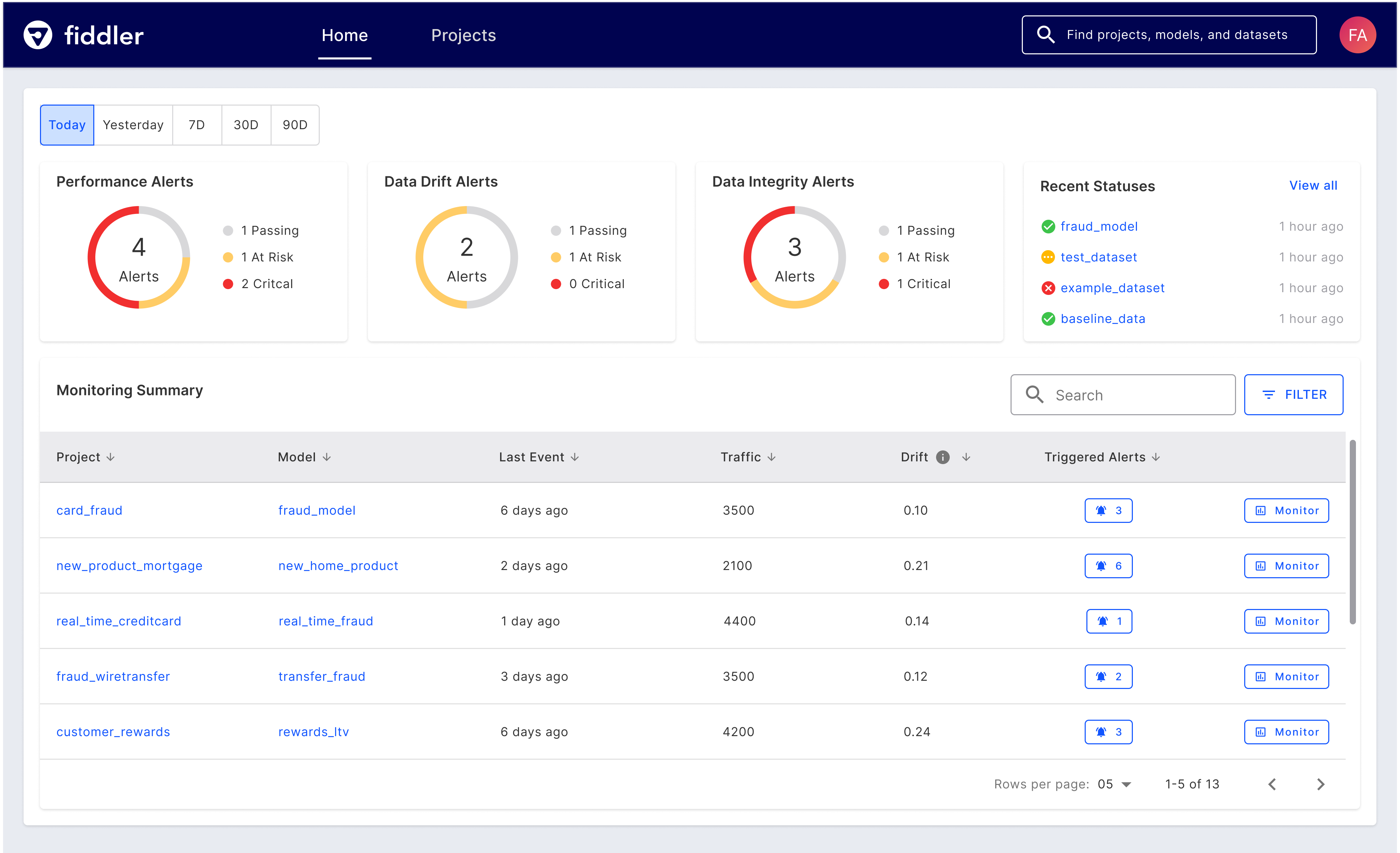
Watch Fiddler’s centralized UI with powerful monitoring dashboards
Flexible APIs
Flexible APIs accelerate onboarding to Fiddler for model monitoring. Monitoring and XAI APIs are decoupled allowing customers focused only on model monitoring use cases to onboard models quickly without needing anything beyond their baseline dataset. Customers can start publishing their production events after registering their baseline dataset, allowing for the Fiddler MPM platform to easily monitor for drift, data integrity, and performance issues.
We are hosting a demo-driven webinar to show these major upgrades on Tuesday, August 16, 2022 at 10am PST / 1pm ET. Save your seat for the upcoming webinar to learn more!