Our Generative AI Meets Responsible AI summit included a great panel discussion focused on the best practices for responsible AI. The panel was moderated by Fiddler’s own Chief AI Officer and Scientist, Krishnaram Kenthapadi and included these accomplished panelists: Ricardo Baeza-Yates (Director of Research, Institute for Experiential AI Northeastern University), Toni Morgan (Responsible Innovation Manager, TikTok), and Miriam Vogel (President and CEO, EqualAI; Chair, National AI Advisory Committee). We rounded up the top three key takeaways from this panel.
TAKEAWAY 1
ChatGPT and other LLMs may exacerbate the global digital divide
Ricardo Baeza-Yates showed how inaccurate ChatGPT can be by demonstrating inaccuracies found in his biography generated by ChatGPT 3.5 and GPT-4, including false employment history, awards, and incorrect birth information, notably that GPT 3.5 states that he is deceased. Despite an update to GPT-4, the speaker notes that the new version still contains errors and inconsistencies.
He also identified issues with translations into Spanish and Portuguese, observing that the system generates hallucinations by providing different false facts and inconsistencies in these languages. He emphasized the problem of limited language resources, with only a small fraction of the world's languages having sufficient resources to support AI models like ChatGPT. This issue contributes to the digital divide and exacerbates global inequality, as those who speak less-supported languages face limited access to these tools.
Additionally, the inequality in education may worsen due to uneven access to AI tools. Those with the education to use these tools may thrive, while others without access or knowledge may fall further behind.
TAKEAWAY 2
Balancing automation with human oversight is key
Toni Morgan shared the following insights from their work at Tiktok. AI can inadvertently perpetuate biases and particularly struggles with context, sarcasm, and nuances in languages and cultures,, which may lead to unfair treatment of specific communities. To counter this, teams must work closely together to develop systems that ensure AI fairness and prevent inadvertent impacts on particular groups. When issues arise with the systems' ability to discern between hateful and reappropriated content, collaboration with content moderation teams is essential. This requires ongoing research, updating community guidelines, and demonstrating commitment to leveling the playing field for all users.
Community guidelines are needed and helpful that cover hate speech, misinformation, and explicit material. However, AI-driven content moderation systems often struggle with understanding context, sarcasm, reappropriation, and nuances in different languages and cultures. Addressing these challenges necessitates diligent work to ensure the right decisions are made in governing content and avoiding incorrect content decisions.
Balancing automation with human oversight is a challenge that spans across the industry. While AI-driven systems offer significant benefits in terms of efficiency and scalability, relying solely on them for content moderation can lead to unintended consequences. Striking the right balance between automation and human oversight is critical to ensuring that machine learning models and systems minimize model bias while aligning with human values and societal norms.
TAKEAWAY 3
Achieving responsible AI requires internal accountability and transparency
Miriam Vogel offered these tips: Ensure accountability by designating a C-suite executive responsible for major decisions or issues, providing a clear point of contact. Standardize processes across the enterprise to build trust within the company and among the general public. While external AI regulations are crucial, companies can take internal steps to communicate the trustworthiness of their AI systems. Documentation is a vital aspect of good AI hygiene, including recording testing procedures, frequency, and ensuring the information remains accessible throughout the AI system's lifespan. Establish regular audits to maintain transparency about testing and its objectives, as well as any limitations in the process. The NIST AI Risk Management Framework, released in January, offers a voluntary, law-agnostic, and use case-agnostic guidance document developed with input from global stakeholders across industries and organizations. This framework provides best practices for AI implementation, but with varying global standards, the program aims to bring together industry leaders and AI experts to further define best practices and discuss ways to operationalize them effectively.
We asked the audience whether or not they had begun building out a responsible AI framework and got the following response:
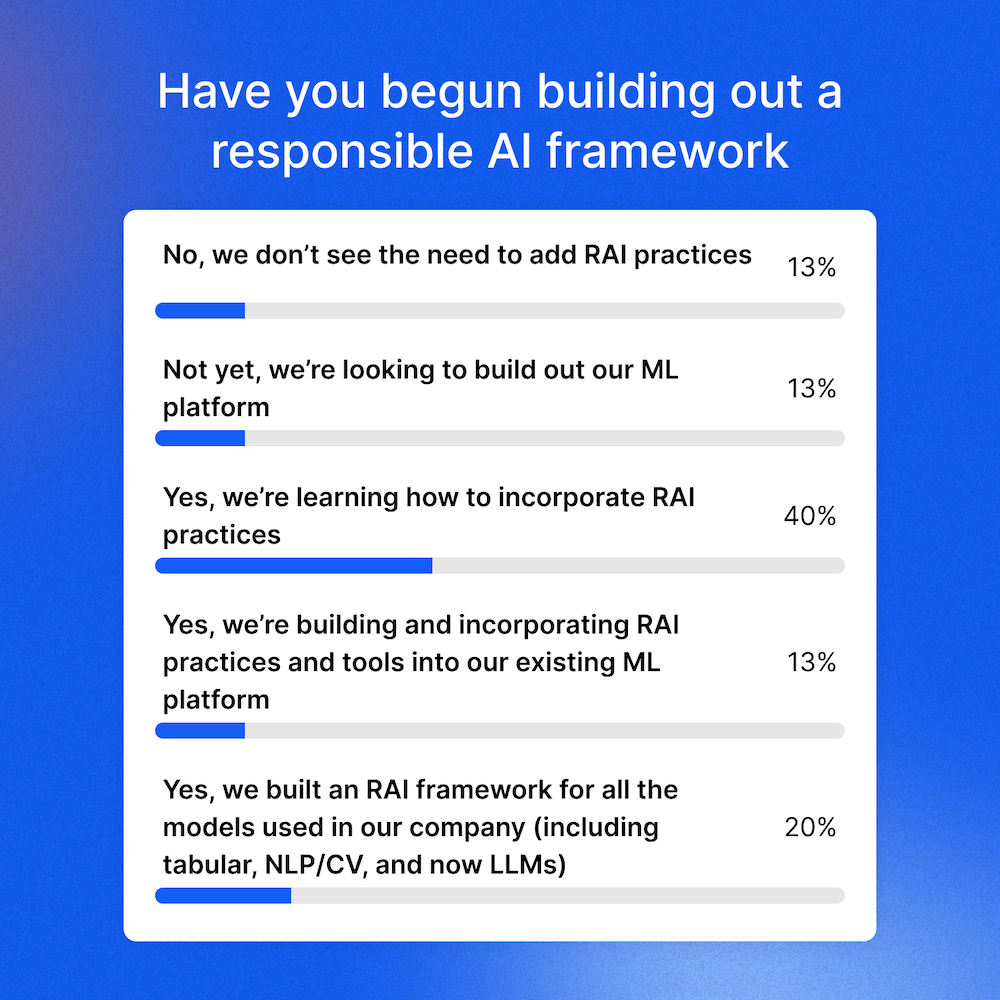
We then asked the audience where they were in their AI journey:

Watch the rest of the Generative AI Meets Responsible AI sessions.